Welcome back to our exploration of AI’s impact on Procurement. If you missed Part 1, which covered the basics of AI in Procurement and the ground-breaking role of GenAI, make sure to catch up. Now, we dive deeper into Machine Learning and Predictive Analytics, demonstrating their pivotal roles in refining strategic sourcing and supplier management.
Let’s talk about Recommender Systems like Spotify.
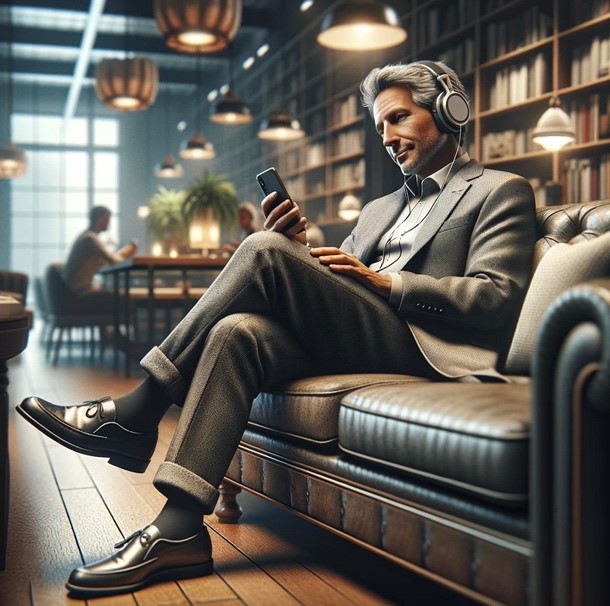
Picture this: You’re kicking back, your headphones are on, and you are listening to your favourite anthems on Spotify—from INXS to Midnight Oil classics. Ever wonder how Spotify knows what you want to hear next? That’s Machine Learning (ML) and Predictive Analytics at work, crafting that perfect playlist tailored just for you.
Now, imagine applying this personalised, insightful approach to supplier management and selection.
Machine Learning in Procurement is instrumental in analysing historical data (like your Spotify history). For example, let’s think about supplier performance. ML can scrutinise metrics such as delivery times, product quality and compliance rates, gaining insights into supplier behaviour and performance trends by processing this historical data.
This learning isn’t just about understanding data pre-determinedly; it involves identifying patterns and relationships within the data that weren’t explicitly programmed into the system.
By analysing historical data, ML algorithms can identify patterns – for example, which suppliers consistently meet delivery timelines or which suppliers tend to be repeat poor-quality offenders. It then uses these patterns to make predictions or decisions, such as identifying which suppliers will likely be reliable. A key feature of ML is that these algorithms can improve their predictions or decision-making over time as they process more data. So, as new supplier performance data comes in, the ML system refines its understanding of what characterises a reliable or high-risk supplier.
This goes beyond traditional data processing or rule-based AI. In rule-based systems, every possible scenario and response needs to be pre-programmed. ML, by contrast, can develop its own ‘rules.’
The predictive aspect of AI comes into play when these learned patterns and insights are used to forecast future outcomes. For instance, AI can predict which suppliers are likely to be most reliable or which may present risks based on their historical performance data.
Use Case: Supplier Performance
Consider a scenario where a procurement team evaluates suppliers for a critical component. Machine Learning algorithms first analyse data from various suppliers over time, capturing and learning from metrics like delivery timeliness, quality and compliance with contractual obligations. This learning phase creates a foundation of knowledge about each supplier’s past. In the prediction phase, AI utilises these insights to forecast the future reliability of these suppliers. It might predict that Supplier A, despite having occasional delays, offers the best quality and compliance history, making them a safer choice for critical components. Simultaneously, predictive analytics could flag Supplier B as a high-risk choice due to a pattern of declining quality in their products.
This comprehensive approach, blending ML’s learning capabilities with predictive analytics, empowers procurement teams with a more nuanced, forward-looking strategy in supplier selection and risk management, ensuring more resilient and efficient procurement operations.
AI & Decisioning
AI is not just an assistant but a catalyst transforming decision-making processes from the ground up.
Siemens‘ innovative use of AI showcases a model of using AI to support decision-making. AI’s role has been identifying alternative suppliers, understanding and predicting challenges within the global value chain, and integrating real-time supply chain intelligence. This approach leverages AI to provide in-depth analyses and insights, informing human decision-makers in critical areas such as supplier selection and risk management.
AI is making decision-making more efficient, accurate, and tailored to organisations’ strategic needs. But how does this technology decide, and what does this mean for the future of Procurement?
Decision-making can be categorised into fully automated and partially automated procedures. Let’s use the example of approving purchasing orders to illustrate the difference.
Use Case: Approving Purchase Orders
Fully Automated Decisioning is a system where decisions, such as approving purchase orders, are instantaneously made based on predefined rules. This could be on low-spend, low-risk purchases. This method offers significant speed advantages, enabling immediate decision-making. Consistency is a key benefit here, as each decision, from the first to the thousandth purchase order, adheres to the same set of rules, minimising the possibility of human error. This approach is especially beneficial when dealing with large transactions, eliminating the need to increase staff proportionally. However, this method’s rigidity may overlook unique scenarios and rely heavily on the precision and relevance of the established rules. Should these rules become outdated or inherently flawed, the effectiveness of the decision-making process could be at risk.
Partially Automated Decisioning, conversely, offers a more balanced approach, combining AI’s efficiency with human judgment. In this setup, procurement requests are initially processed using automated criteria. However, unlike the fully automated method, scenarios or requests that meet predefined criteria are routed for human review, guided by their specific delegation authorities. This dual-layered approach ensures that more complex or unusual cases are subjected to human scrutiny, thus providing a more adaptable and nuanced decision-making process. The benefit here is a marriage of speed and ability. Applications are processed rapidly, but a layer of human oversight ensures accuracy and discretion. This collaborative approach also allows various stakeholders to be part of the decision, fostering a more inclusive and informed decisioning process. However, it’s worth noting that this method does lean on stakeholder availability. And, as with any human intervention process, there are potential inconsistencies or errors.
Are you thinking about AI as a contributor to decision-making?
Reflecting on the balance between automation and human oversight can reveal new pathways to decision-making. To facilitate this exploration, consider the following thought starters:
- Integration in Decision Processes: How can AI streamline procurement decisions from simple to complex, such as spend data categorisation, with humans performing final validations? Integrating AI helps sift through vast datasets, allowing procurement professionals to focus on strategic decision-making and analysis.
- Automation vs. Human Insight: In which procurement areas should AI take the lead, and where is human insight indispensable? For example, AI might analyse supplier performance metrics, while humans could address nuanced supplier relationships and negotiations.
- Risk Management in AI Decisions: How do we mitigate risks in automated procurement decisions, particularly in contract analysis or supplier selection? Identifying and implementing checks and balances ensures AI’s reliability and aligns with procurement standards.
- Enhancing Decision Accuracy: How does AI improve the accuracy of forecasting demand or assessing supplier risk? Utilising AI for predictive analysis in procurement can lead to more informed, data-driven decisions, enhancing operational efficiency.
- Future of AI in Procurement Decisioning: What advancements in AI are on the horizon that could further transform procurement decision-making? Staying ahead of technological trends allows procurement to improve and innovate processes continuously.
Reflecting on these questions encourages consideration of AI’s role in decision-making, focusing on practical implementation and the balance between technology and human expertise.
It’s evident that these AI-driven tools are not just futuristic concepts but are actively reshaping how we make decisions today. By leveraging AI’s predictive capabilities, procurement professionals can anticipate challenges and opportunities, making (even more) informed decisions that drive value and efficiency.
In our upcoming final instalment, we will discuss negotiation bots, tackle the ethical considerations of AI, and discuss the broader socioeconomic impacts of technological integration in Procurement. Stay tuned as we explore how AI continues redefining procurement boundaries, promising a future where technology and human expertise converge.
About the author:
Antonia Macrides (Connect on LinkedIn)
Antonia established Australia’s first boutique procurement recruitment firm, a pioneer in leveraging technology to map and measure human capability in the industry. She has since interviewed over 10,000 Procurement Professionals and has used technology and AI to assess the skills, attitudes, and aptitudes of more than 1,000,000 multi-sector individuals. Antonia has uniquely combined her technological expertise, psychological insights and a keen interest in behavioural economics to give organisations the ‘human edge’ in procurement. Her academic background in research, psychology, theology, and AI certification has equipped her with a comprehensive understanding of the human factors that drive procurement success.